Introduction to AI Ethics in B2B
The emergence of artificial intelligence (AI) has significantly transformed the B2B landscape, providing businesses with innovative tools and methods for decision-making. However, as the implementation of AI solutions becomes more commonplace, the ethical considerations surrounding their use have gained prominence. AI ethics in the context of business-to-business transactions is critical due to the implications that biased algorithms and decision-making processes can have on various aspects of a company’s operations.
One key reason for prioritizing ethical considerations in AI is the impact of biased decision-making on a company’s reputation. In an era where businesses are held accountable for their practices, any perceived or real bias in AI systems can lead to public backlash. This negative perception can erode trust among customers, partners, and stakeholders, ultimately affecting a company’s bottom line. Thus, ensuring that AI systems operate with a high ethical standard is not only a moral imperative but also a strategic necessity for companies aiming to maintain competitive advantage.
In addition to reputational risks, businesses must also consider the legal ramifications of relying on biased AI decision-making. As regulatory frameworks around AI continue to evolve, companies could face significant penalties and legal challenges if their AI systems result in discrimination or unfair treatment of clients or employees. Compliance with emerging laws and established ethical standards will be essential for businesses seeking to navigate the landscape of AI responsibly.
Furthermore, ethical AI practices enhance stakeholder trust, as stakeholders increasingly prioritize transparency and fairness in business operations. By embedding ethical principles in their AI strategies, organizations can foster stronger relationships with all parties involved, including customers, employees, and investors. This trust is essential for long-term success and stability in a competitive market. Thus, addressing AI ethics is fundamental for B2B enterprises as they leverage technology to drive growth and innovation.
Understanding Bias in AI
Bias in artificial intelligence (AI) refers to systematic errors that result in unfair outcomes, which can occur at various stages of AI development and deployment. Understanding the nature of this bias is crucial, especially in B2B contexts where decisions can significantly impact operations, resources, and organizational relationships. Bias in AI can be generally categorized into three types: data bias, algorithmic bias, and human bias.
Data bias arises from the datasets used to train AI systems. If the data is unrepresentative or skewed toward particular demographics, the AI system will reflect these inequalities. For example, in a B2B recruitment AI that relies on historical hiring data, if the previous hires predominantly favor one gender or ethnicity, the AI may preferentially select similar candidates, perpetuating unfair hiring practices. Such instances exemplify how data bias can have far-reaching implications, particularly in talent acquisition.
Algorithmic bias is linked to how the AI’s underlying algorithms process data and generate outcomes. Even if the dataset is unbiased, the choice of algorithms or their parameters can inadvertently produce biased results. For instance, an AI used for credit scoring in B2B finance may utilize formulas that base decision-making on factors that historically favor certain industries or company sizes, leading to discrimination against smaller firms or startups that may have equally viable proposals.
Lastly, human bias plays a critical role in shaping AI systems. The design choices and assumptions made by developers can introduce personal biases into the system. For instance, if developers unwittingly embed their own biases regarding what constitutes a ‘successful’ business, the AI may favor companies that align with these subjective criteria, potentially overlooking innovative enterprises that do not fit the preconceived mold. Recognizing these biases is the first step toward mitigating their effects in AI-driven decision-making processes.
Sources of Bias in B2B AI Systems
Artificial intelligence (AI) systems in B2B contexts can be prone to various forms of bias, which can compromise decision-making processes and ultimately affect business outcomes. Understanding the sources of this bias is crucial for businesses seeking to implement AI responsibly. One primary source of bias is the method of data collection. If the data gathered is not representative of the target population or scenario, the AI system trained on this data is likely to yield skewed results. For instance, if customer data predominantly includes information from one geographic region, the AI may underperform in different areas, leading to misinformed decisions.
Lack of diversity in training datasets is another significant contributor to bias within AI systems. Many B2B organizations utilize historical data to train their algorithms, which might reflect existing inequalities or prejudices. If the training dataset comprises limited demographic groups or fails to include voices from various backgrounds, the AI system may replicate these biases, leading to unjust criteria for assessing clients or suppliers. Thus, it is essential to ensure that data used for training AI incorporates a broad spectrum of perspectives and experiences.
Moreover, biases inherent in the algorithms themselves can also lead to biased outcomes in AI systems. Algorithms are designed based on specific assumptions and logic, which can inadvertently reflect the biases of their creators. This element of human bias can seep into programming, thereby impacting decision-making. Consequently, businesses must engage in thorough audits of the algorithms and their underlying logic to identify and mitigate potential biases.
Recognizing the sources of bias in B2B AI systems is the first step toward creating fairer and more effective AI tools. Addressing these biases proactively can significantly enhance the quality and equity of the decision-making processes that businesses depend upon.
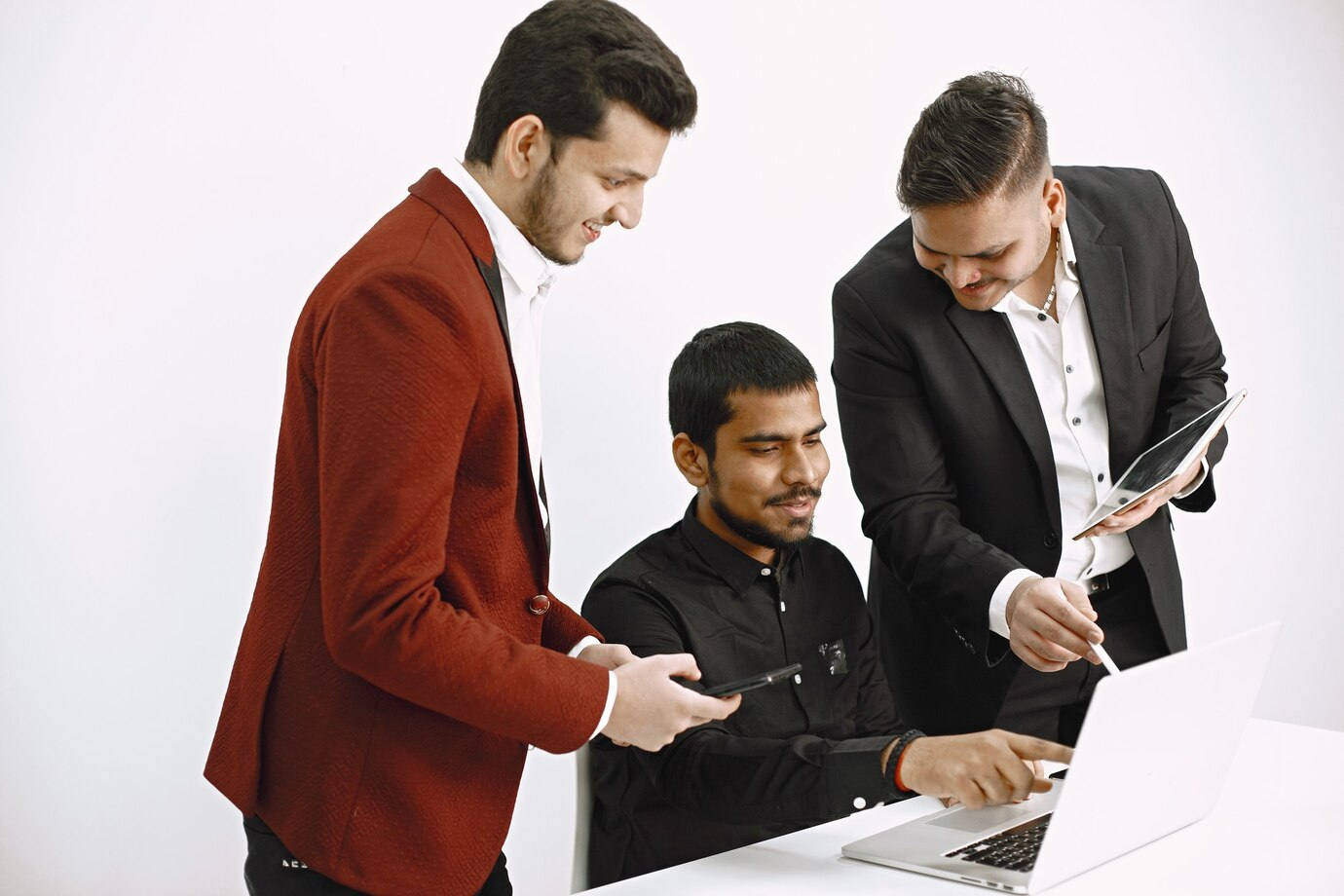
Consequences of Bias in Decision-Making
In the realm of B2B interactions, biased decision-making can have far-reaching and detrimental consequences. One of the most immediate impacts is on business performance. When decisions are influenced by bias, they often fail to reflect the true needs of the market or the potential of the stakeholders involved. This misalignment can lead to suboptimal product development, ineffective marketing strategies, and ultimately, a decline in competitive advantage. Companies that do not mitigate bias may find themselves outperformed by competitors who prioritize data-driven decision-making.
In addition to impaired performance, organizations also face significant legal risks associated with biased practices. Decisions that favor certain groups over others can result in discrimination claims and regulatory penalties. For instance, recruitment processes that unintentionally favor a specific demographic may lead to accusations of unfair hiring practices. Such legal challenges not only incur additional costs but also drain company resources that could be better allocated elsewhere.
The damage to reputation resulting from biased decision-making is another critical concern. A company identified as biased may struggle to attract customers, partners, or even potential employees, as market sentiment increasingly steers toward ethical business practices. In today’s digital age, negative perceptions can spread rapidly, leading to long-lasting impacts on a company’s public image.
Furthermore, customer trust plays a pivotal role in the success of any B2B relationship. When clients perceive bias in an organization’s decision-making, their confidence in the business erodes. This decline in trust can significantly affect customer retention and loyalty, which are vital for sustained success. Stakeholders expect ethical responsibilities to be upheld, and failure to do so not only jeopardizes relationships but also affects overall market stability. An examination of case studies involving companies that faced backlash due to biased decisions illustrates the profound ripple effects of these issues. Therefore, addressing bias is not merely a best practice but an essential aspect of responsible business conduct.
Frameworks for Ethical AI Development
As the integration of artificial intelligence (AI) across various business sectors continues to evolve, the emphasis on ethical AI development has similarly gained momentum. Implementing frameworks and guidelines that address ethical considerations is essential for organizations aiming to minimize bias in their decision-making processes. One of the most recognized approaches is the adoption of the EU’s Artificial Intelligence Act, which lays the groundwork for the development and use of AI systems that respect fundamental rights, ensuring accountability and transparency.
In addition to regulatory standards, several organizations advocate for specific ethical frameworks that can guide businesses in developing responsible AI. For instance, the IEEE Global Initiative on Ethics of Autonomous and Intelligent Systems has provided extensive resources, including the “Ethically Aligned Design” document, which outlines principles for AI development aimed at fostering trustworthiness and inclusivity. Such frameworks encourage businesses to consider the ethical implications of their technology, leading to AI systems that are designed with fairness as a priority.
Furthermore, incorporating best practices into the AI development lifecycle can significantly reduce the risk of bias. Organizations are encouraged to engage in multi-disciplinary collaboration, bringing together individuals from different backgrounds and expertise to participate in the AI design process. This diverse input can provide a more comprehensive perspective, highlighting potential areas of bias that may not be apparent to a homogenous development team. Regular audits and assessments of AI systems can also ensure that they remain aligned with ethical standards throughout their deployment.
By prioritizing ethical frameworks and adhering to best practices, businesses can drive responsible AI development. This, in turn, not only mitigates bias but also fosters a culture of accountability and trust, reinforcing the integrity of AI applications in the business landscape.
Strategies to Mitigate Bias in AI Decision-Making
Addressing bias in artificial intelligence is pivotal for businesses aiming for fairness and ethical standards in decision-making processes. The first strategy involves enhancing data diversity. It is essential for organizations to ensure that the datasets used to train AI models reflect diverse demographics, including gender, race, and socioeconomic factors. A representative dataset reduces the risk of biased outcomes and enhances the model’s accuracy in a broader context. Businesses should actively seek diverse data sources and employ techniques such as synthetic data generation if adequate real-world data is unavailable.
Another vital strategy is the implementation of regular audits. By systematically assessing AI systems for bias, organizations can identify potential pitfalls and take corrective measures proactively. These audits should examine not only the outcomes of AI decisions but also the processes that generated these outcomes. Utilizing third-party evaluators can bring an unbiased perspective to the auditing process, thus allowing for more transparent evaluation and risk mitigation.
Promoting interdisciplinary teams is equally crucial. Diverse teams that include not only data scientists but also ethicists, sociologists, and domain-specific experts can provide multifaceted insights that help illuminate potential biases in AI algorithms. This varied approach leads to more comprehensive analyses and encourages discussions around ethical considerations, thus enriching the decision-making process itself.
Lastly, employing fairness-aware algorithms presents a compelling solution. Many modern AI frameworks now provide options to integrate fairness constraints directly into the AI development lifecycle. These algorithms are designed to identify and counteract biased tendencies automatically, providing a necessary tool in the effort to create equitable systems. Businesses should thoroughly research and incorporate these cutting-edge methodologies to advance their AI initiatives responsibly.
The Importance of Human Oversight in AI Decision-Making

As artificial intelligence (AI) systems continue to evolve and play a pivotal role in decision-making processes, particularly in the business-to-business (B2B) landscape, human oversight becomes increasingly essential. The integration of human judgment serves as a critical check on technological systems, especially in identifying and correcting potential biases that may arise. Bias in AI can stem from various sources, including skewed training data or misaligned algorithms, which can lead to unfair outcomes. Therefore, human oversight is integral in ensuring that ethical considerations remain a priority.
Human intervention is particularly important in evaluating AI models and the data they rely upon. By incorporating diverse perspectives into the development and deployment of AI systems, organizations can more effectively detect and mitigate biases. This involves not only monitoring the data inputted into AI systems but also assessing the output to ensure that it aligns with ethical standards and organizational values. Regular audits conducted by human experts can identify discrepancies in AI decision-making that may have been overlooked during the initial design phase.
Furthermore, human oversight encourages transparency within AI-driven processes. Stakeholders need to understand the rationale behind AI decisions, which can often be obscured by the complexity of algorithms. When human experts analyze and explain these decisions, it fosters accountability and helps to build trust among users and clients. By bridging the gap between technology and human values, businesses can leverage AI to enhance efficiency while maintaining a commitment to ethical practices.
Ultimately, human oversight acts not only as a safeguard against bias but also as a means to promote a culture of ethical decision-making within organizations. By prioritizing human involvement in AI decision processes, businesses can navigate the intricacies of technology while upholding their ethical obligations, ensuring that AI serves as a force for good in the B2B sector.
Building a Culture of Ethical AI in Your Organization
Establishing a culture of ethical AI within an organization is paramount for ensuring that artificial intelligence technologies are leveraged responsibly. As AI increasingly informs business decisions, organizations must integrate ethical considerations into their core practices. This begins by setting clear expectations around the ethical use of AI, with policies that emphasize fairness, accountability, and transparency. By doing so, businesses can mitigate the risk of bias in decision-making.
Training employees on AI ethics is another critical component of cultivating this culture. Workshops and training sessions should cover essential topics such as understanding algorithmic bias, identifying potential ethical dilemmas, and implementing best practices in AI deployment. This education not only cultivates awareness among employees but also empowers them to challenge unethical practices and contribute to responsible AI initiatives. Tailoring training programs to different departments within the organization can ensure that the ethical implications of AI are relevant and specific to various functions.
Moreover, encouraging open discussions about AI’s societal implications is vital for fostering an ethical culture. Organizations should promote an environment where employees feel comfortable raising concerns or suggesting improvements regarding AI systems. This can be facilitated through regular forums, feedback sessions, or brainstorming workshops that invite diverse perspectives. Additionally, incorporating cross-functional teams can help capture varied viewpoints and enhance the overall discourse around ethical AI practices.
Ultimately, a proactive approach to building a culture of ethical AI not only enhances decision-making processes but also reinforces the organization’s reputation in the marketplace. By prioritizing ethics in AI, businesses can lead by example and contribute positively to societal values, ensuring that technological advancements are beneficial and equitable.
Conclusion and Call to Action
As artificial intelligence continues to shape the landscape of business-to-business (B2B) interactions, the potential for bias in decision-making cannot be overlooked. Bias in AI systems can lead to unfair practices, distorted outcomes, and reputational damage for companies that fail to address these issues proactively. It is imperative for businesses to understand the sources and impacts of bias in AI, particularly as they implement these technologies within their operational frameworks.
This blog has highlighted the importance of recognizing how biased data and algorithmic processes can affect outcomes in B2B settings. To mitigate these risks, companies must adopt comprehensive strategies that include regular audits of their AI systems, the utilization of diverse data sets, and involvement from interdisciplinary teams in the design and evaluation of AI models. By prioritizing ethical considerations in AI deployment, businesses can enhance accountability and transparency while cultivating a culture of fairness.
Furthermore, companies should invest in ongoing education and training for employees to ensure they are well-informed about the ramifications of bias in AI. This not only promotes ethical conduct but also fosters an organizational ethos centered on inclusivity and responsibility. It is essential that businesses remain vigilant and proactive in monitoring their AI systems for potential biases, continuously seeking to improve and refine their practices.
In light of these considerations, we encourage organizations to commit to the principles of ethical AI in their decision-making processes. The future of business technology depends on our collective ability to foster a more equitable and trustworthy environment. By taking strides towards addressing bias in AI, businesses can not only protect their interests but also contribute positively to the broader B2B ecosystem. Together, let us pave the way for an ethical future in AI-driven decision-making.