In recent years, the use of Artificial Intelligence (AI) has skyrocketed across industries, transforming business operations, optimizing workflows, and enhancing decision-making processes. For B2B companies, leveraging AI has become a crucial competitive advantage. However, one challenge has always loomed over AI applications: data scarcity. Many businesses struggle to access high-quality, relevant, and privacy-compliant data, which limits their ability to fully harness the power of AI. This is where synthetic data comes in as a game-changing solution.
In this article, we’ll dive deep into the concept of synthetic data, its role in the AI ecosystem, and why it is considered the ultimate secret weapon for B2B businesses looking to elevate their AI strategies. Whether you’re a startup or an established enterprise, synthetic data holds transformative potential for your AI-driven initiatives.
What is Synthetic Data?
At its core, synthetic data is artificially generated data that mimics real-world data in terms of structure, distribution, and statistical properties. Unlike traditional data, which is collected through real-world processes such as transactions, user interactions, or sensors, synthetic data is created using algorithms, machine learning models, and simulations. It replicates real-world data in a way that is both realistic and diverse but without using actual sensitive information or real-world data sources.
Synthetic data can take various forms, such as:
- Images and Videos: Generated using computer graphics or simulation software, often used in training computer vision models.
- Text: Created using natural language processing models to mimic real-world conversations, reviews, or other textual data.
- Time-Series Data: Simulated data that represents sequential events or trends over time, often used in forecasting and predictive modeling.
- Tabular Data: Generated for structured datasets that represent business operations, such as sales data, inventory data, or financial transactions.
The primary advantage of synthetic data is that it allows businesses to create vast amounts of data for training AI models without the constraints of data collection, privacy issues, or cost barriers.
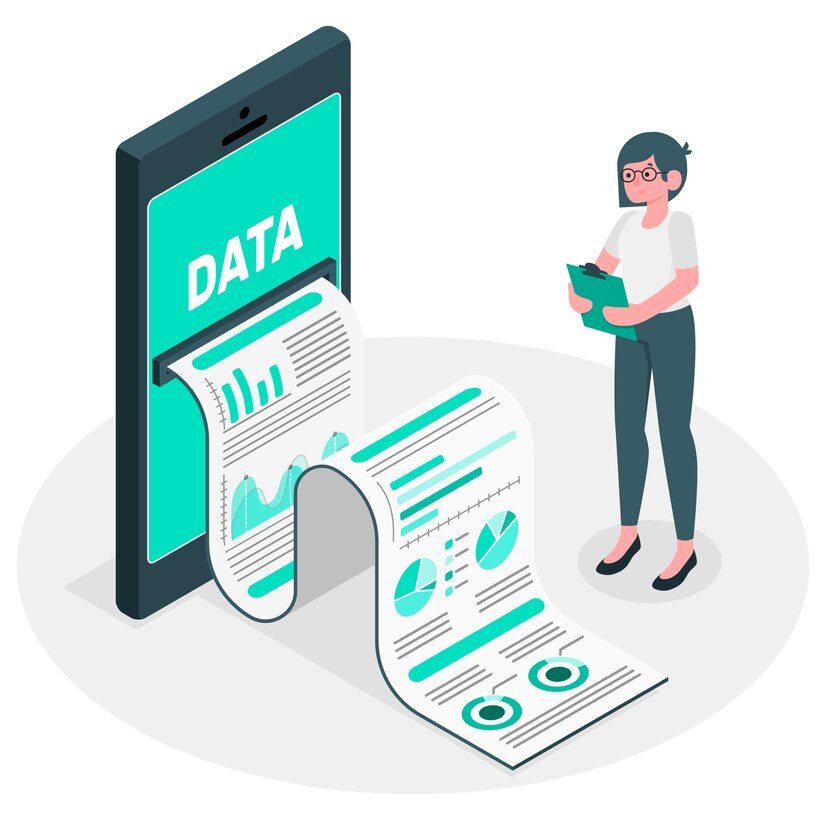
Why Synthetic Data Matters for B2B AI
B2B companies are increasingly adopting AI technologies to improve efficiency, reduce costs, and drive innovation. However, to develop accurate and reliable AI models, high-quality data is essential. In this context, synthetic data provides multiple benefits for B2B organizations seeking to optimize their AI investments. Below are some key reasons why synthetic data is a valuable asset for businesses:
1. Solving the Data Scarcity Problem
In many industries, obtaining enough relevant and high-quality data can be a daunting task. For instance, in sectors like healthcare, finance, and manufacturing, access to real-world data is often limited due to privacy regulations, proprietary concerns, or the sheer cost of data collection.
Synthetic data provides a way to overcome these barriers. It can be generated in abundance, covering a wide range of scenarios and use cases that may be difficult or expensive to collect in the real world. This enables B2B companies to develop more robust AI models by filling the data gaps that would otherwise slow down development and deployment.
2. Mitigating Privacy and Security Risks
Data privacy is a critical concern, particularly for industries that handle sensitive information such as healthcare or finance. Real-world data can contain personally identifiable information (PII) or other confidential data that is subject to stringent regulations like the GDPR, CCPA, or HIPAA.
With synthetic data, B2B companies can avoid these privacy concerns. Since synthetic data is artificially generated and doesn’t contain any real personal information, it can be used to train AI models without violating privacy regulations. This reduces the risk of data breaches or non-compliance, ensuring that businesses remain on the right side of the law.
3. Accelerating AI Model Development
Training AI models requires a substantial amount of data. The more data an AI system is trained on, the more accurate and reliable it becomes. However, collecting and cleaning real-world data is time-consuming and costly.
Synthetic data allows businesses to bypass these challenges. By generating large datasets rapidly, companies can accelerate the training process, reducing the time-to-market for AI solutions. This speed can be a significant advantage, especially in competitive B2B environments where agility and time-to-market are crucial factors for success.
4. Enhancing Model Generalization
One of the key challenges in AI model development is ensuring that models generalize well to new, unseen data. If an AI system is only trained on a narrow dataset, it may perform well on similar data but fail when exposed to new or diverse scenarios.
Synthetic data enables B2B companies to create highly diverse datasets that reflect a wide range of conditions, edge cases, and rare events. By training AI models on these varied datasets, businesses can improve the generalization of their models, making them more robust and adaptable to real-world situations.
5. Overcoming Bias in Data
Bias in AI models is a significant concern. If the data used to train AI systems is biased, the models will inherit those biases, leading to unfair or discriminatory outcomes. This can be particularly problematic in sectors like hiring, lending, or criminal justice, where biased AI decisions can have serious consequences.
Synthetic data can help mitigate bias by creating balanced datasets that are free from historical prejudices. By generating diverse and representative datasets, B2B companies can reduce the risk of bias in their AI models, ensuring that their systems make fair and equitable decisions.
How Synthetic Data Fuels Innovation in B2B AI Applications
Synthetic data is transforming how B2B companies approach AI development, unlocking new opportunities for innovation and growth. Let’s explore some key areas where synthetic data is driving AI advancements in the B2B sector.
1. AI in Healthcare
Healthcare organizations are increasingly adopting AI for tasks such as diagnostics, treatment recommendations, and drug discovery. However, the availability of high-quality, annotated medical data is often limited due to privacy concerns and data sharing regulations.
By using synthetic data, healthcare organizations can train AI models on diverse datasets that simulate real-world medical scenarios without violating patient confidentiality. Synthetic data can be used to create medical images, patient records, or clinical trial data, allowing AI systems to learn from a broader range of situations and improving their performance in real-world applications.
2. AI in Financial Services
In the financial sector, AI is widely used for fraud detection, risk assessment, algorithmic trading, and customer service automation. However, financial institutions often face challenges in accessing enough data to train accurate AI models. Moreover, the use of real financial data can raise privacy concerns and regulatory hurdles.
Synthetic financial data can be generated to simulate various market conditions, customer behaviors, and transaction patterns. This enables financial institutions to train AI models on vast amounts of data while ensuring compliance with data privacy regulations. Synthetic data can also help financial institutions test their models under various stress scenarios, improving their resilience and adaptability.
3. AI in Manufacturing and Logistics
Manufacturers and logistics companies are using AI to optimize supply chains, predict equipment failures, and improve production efficiency. However, collecting real-world data from sensors and machines can be costly and time-consuming.
Synthetic data can be used to simulate sensor readings, machine conditions, and supply chain scenarios, allowing manufacturers to train AI models without the need for extensive real-world data collection. This can help optimize production schedules, predict maintenance needs, and improve overall operational efficiency.
4. AI in Retail and E-commerce
In the retail and e-commerce sectors, AI is used for customer personalization, demand forecasting, inventory management, and recommendation engines. However, retailers often struggle with collecting enough diverse data to train AI models that can understand customer preferences and market trends.
Synthetic data can be used to generate simulated customer interactions, transaction data, and product reviews, helping AI systems make more accurate predictions and recommendations. This enables retailers to deliver personalized experiences to customers while ensuring that their AI models are continuously evolving and improving.
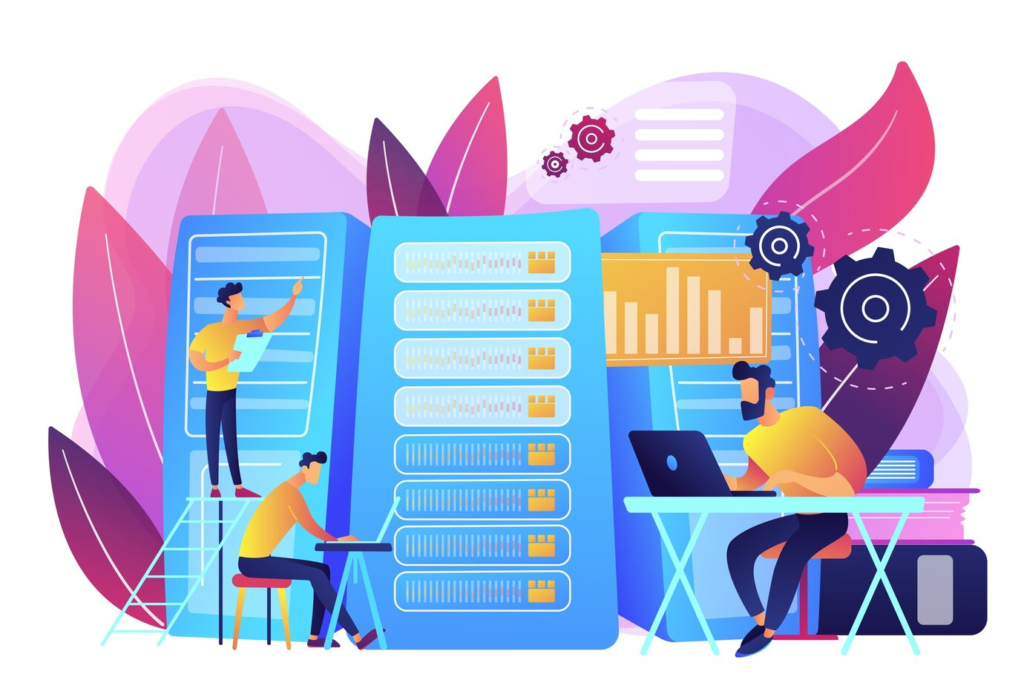
Best Practices for Leveraging Synthetic Data in B2B AI
To fully harness the potential of synthetic data, B2B companies must follow best practices to ensure that their AI models are accurate, reliable, and scalable. Here are some key strategies:
1. Blend Synthetic and Real Data
While synthetic data offers many benefits, it is important to remember that it is not a complete replacement for real-world data. A hybrid approach, where synthetic data is used to augment real data, can often lead to better results. By combining the strengths of both data types, businesses can create more robust and generalizable AI models.
2. Use Domain-Specific Simulations
The quality of synthetic data is highly dependent on how well it reflects the real-world scenarios it aims to simulate. For best results, B2B companies should use domain-specific simulations that closely match their business environment. This ensures that the synthetic data is highly relevant and valuable for AI model training.
3. Ensure Data Quality and Diversity
When generating synthetic data, it’s crucial to focus on data quality and diversity. The more varied and representative the synthetic data is, the more effective it will be in training AI models. Ensure that the synthetic data covers a wide range of scenarios, edge cases, and rare events to help the model generalize effectively.
4. Monitor and Validate Models Continuously
Even with high-quality synthetic data, it’s important to continuously monitor and validate AI models. This ensures that the models are performing as expected and making accurate predictions. Regular validation also helps identify potential biases or flaws in the synthetic data that need to be addressed.
Unleashing the Power of Synthetic Data for AI-Driven Success
In the competitive B2B landscape, AI has become a critical tool for driving business success. However, data challenges—such as scarcity, privacy concerns, and quality issues—often hinder AI model development. Synthetic data emerges as a powerful solution to these challenges, enabling businesses to generate vast amounts of high-quality, privacy-compliant data for training AI models.
By leveraging synthetic data, B2B companies can accelerate AI development, improve model accuracy, and enhance decision-making processes. Whether in healthcare, finance, manufacturing, or e-commerce, synthetic data is opening up new avenues for innovation, helping businesses stay ahead of the curve and unlock the full potential of AI.